In many frontline health systems, poor data quality perpetuates a cycle of mistrust and inefficiency – impacting patient care at scale.
The Data Observation Toolkit (DOT) was created to break this cycle. It’s a community-informed, open-source software and certified Digital Public Good designed to automate data quality checks, detect anomalies, and enhance trust in health data.
DOT was developed by DataKind in partnership with Medic to disrupt the compounding cycle of poor data quality in frontline health systems and drive practical and thoughtful conversations about remediation of inconsistent or problematic data.
An effective health system requires trustworthy data foundations, and systematic data quality assessments are essential for effective healthcare delivery in underserved communities.
In designing DOT, we conducted a research study to understand the most common data quality issues in frontline health systems.
Our study, which was originally published in CHANCE magazine, analyzed data from 7.7 million health encounters from over 4,000 frontline health workers to assess data quality along three dimensions: completeness, accuracy, and timeliness. We found:
- 40% of community health workers recorded implausible body temperature readings, likely due to miscalibrated equipment
- Care pathways frequently contained incomplete data, especially in maternal care – suggesting major gaps in patient follow up
- Frontline health worker activity and performance varied significantly, highlighting a need for more targeted training and support
These findings highlighted that poor data quality isn’t just about individual errors—it’s a systemic issue. Miscalibrated tools, gaps in care documentation, and inconsistent performance patterns all point to structural challenges in how data is captured, managed, and acted upon in frontline health systems.
To address these challenges at scale, we realized that ad hoc interventions—like retraining individuals or spot-fixing datasets—wouldn’t be enough. What’s needed is a systemic, scalable approach that supports better data quality as part of everyday workflows.
This insight shaped our design approach for DOT. Rather than creating a tool for one-time audits or isolated use cases, we focused on building a solution that could:
- Be deployed at the platform level to continuously surface problematic data or care gaps
- Remain modular, open source, and adaptable to diverse technical environments
- Be human-centered, supporting and empowering the frontline health workers who generate this data
With these principles in mind, DOT was designed as a platform-agnostic, community-driven solution that meets the needs of governments, NGOs, and local health organizations around the world.
DOT is already making a difference in Uganda and Lesotho. Stay tuned for more on how DOT works and real-world case studies showing its impact.
Read the full study: Pathways to Increasing Trust in Public Health Data here.
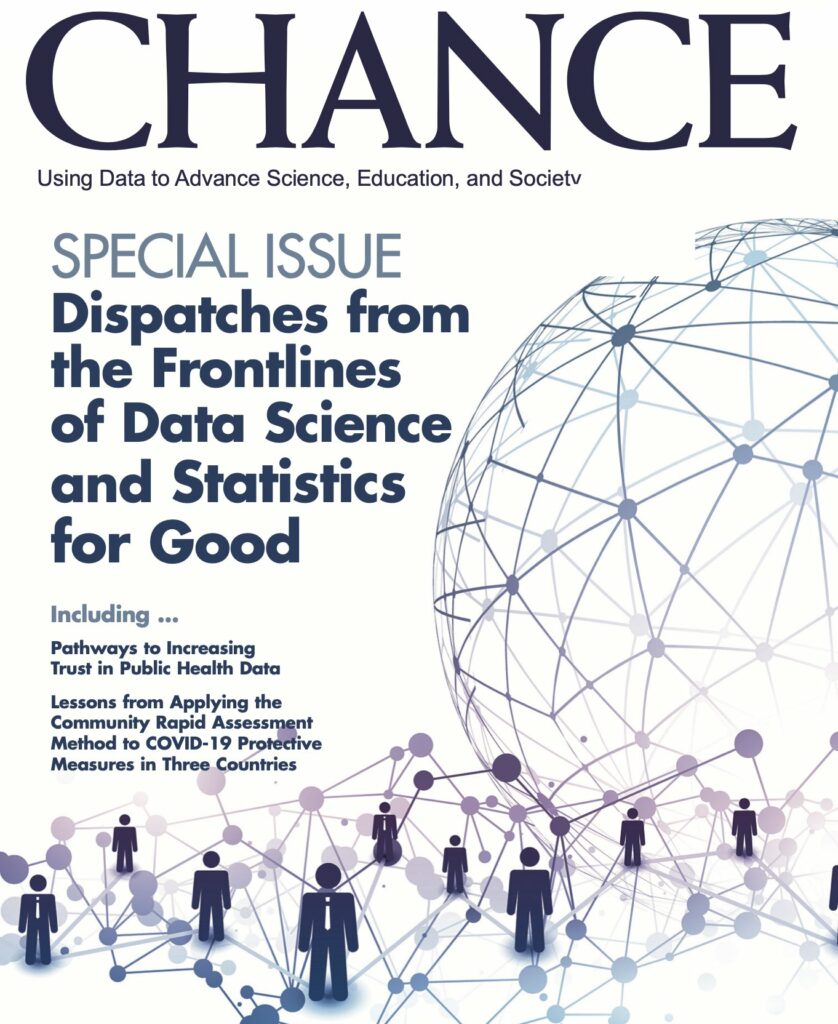
Header image above courtesy of iStock/PaperFox.